Boosting Brand Impact and Influence via Artificial Intelligence
7/18/2024 Greg Rentsch
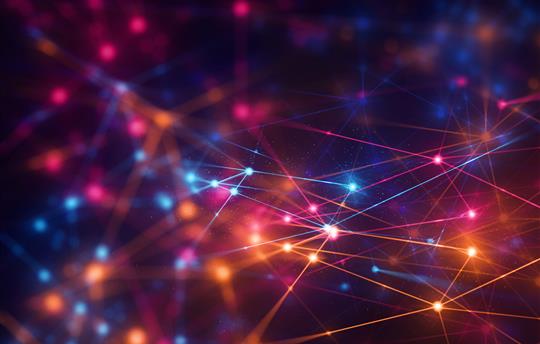
Artificial intelligence (AI) is well-known to the public at large, as many people have now experimented with applications such as Chat GPT for generating content and Dall-E for creating art. What the average consumer may not be aware of, however, is how AI’s applications have become a game changer for marketing research and marketing campaigns.
From custom messaging to predicting downturns and enabling pivots in business strategy, AI has taken data analysis to the next level. AI collects customer data across myriad channels and transforms that data into invaluable insights. Then, AI acts on this information by communicating with customers — hopefully prompting them to make a purchase.
AI, the invaluable influencer
AI is widely used in businesses to aggregate and create algorithms to predict and prompt user behavior. Social media and search engine advertising, ads over email, and e-commerce cart notifications are just some examples of how companies are utilizing AI.
Fast food chain McDonald's, for instance, uses AI-powered digital signs to display menu items that are more likely to sell based on various factors. In addition, the chain uses Dynamic Yield's machine-learning-driven solution to update digital drive-thru menu displays based on time of day, current order selections, restaurant traffic, popularity of menu items, and even weather conditions.
AI has also been making serious strides on the brand influencer side of the equation. Brands like Prada, Dior, and Calvin Klein are using AI-generated influencers, and AI “celebrities” around the globe are proving that they can exert influence on today’s young spenders: Shudu Gram, the world's first digital supermodel; Lu do Magalu and Miquela, with millions of Instagram followers; and Imma, a Japanese virtual influencer.
Boosting impact, tapping emotions
AI can also be used in public relations and communications, as well as for tapping emotional intelligence and seeking influence through it.
RAD AI, for example, is a tool with emotional intelligence that is designed to deliver authentic influencer content programs. It can analyze large data modules about audiences to create detailed personas and then identify the optimal channels for distribution for them. Most importantly, it combines machine learning, natural language processing (NLP), and natural language generation (NLG) to measure authenticity by breaking it down into resonance, behavior, and emotion.
Another application of AI for augmenting marketing strategy comes in the form of revenue acceleration programs like Drift. Drift unites companies with buyers in real-time via chat, video, voice, and email, focusing on the customer lifecycle. Its AI-powered virtual selling assistants (VSAs) engage visitors much like humans would, even responding with spontaneity to mimic an organic conversation. VSAs can provide accurate information and route target accounts to the right sales rep while deflecting support requests away from sales teams.
To lift conversion rates across customer engagement channels, the Persado AI platform utilizes natural language generation, experimental design, and a language base of tagged and scored words. The company works with retailers like Marks & Spencer, The Gap, and Audible, as well as fintech companies like Vanguard Group, to help their customers achieve incremental revenue.
Letterdrop, a content operations platform, helps B2B companies quickly edit and repurpose information to create and distribute more content to drive inbound leads. Featuring an AI-assisted SEO optimizer, Letterdrop can generate email and social-media content from long-form content while offering better insights into what customers want to create appropriate messaging.
Deeper down the AI well
As AI adoption makes further inroads across industries, here are two additional trends to be aware of:
Large language models — The adoption of large language models (LLMs) will impact AI-assisted customer service. By processing vast amounts of text data, these AI systems are capable of understanding and then generating human language. Uses include text generation and content summarization, as well as chatbots, code generation, and even sentiment analysis for customer feedback.
Multimodal AI — Combining conversational AI and visual models, multimodal AI can pull in data from video, images, speech, sound, and text to create in-depth analyses of real-world problems. An example is Google's Multitask Unified Model (MUM), which uses AI to improve the search experience through the T5 text-to-text framework, where search results are based on contextual information from multiple languages. Because MUM is multimodal, it understands information across text and images and will eventually expand to modalities like audio and video.
As companies seek to navigate how they can best utilize AI, they also need to consider parameters of safety, responsibility, privacy, and accuracy, with governments seeking to draw lines to control its unfettered expansion.
Greg Rentsch is RRD's Vice President of Operations and General Manager.